Quants worth following: Judith Gu
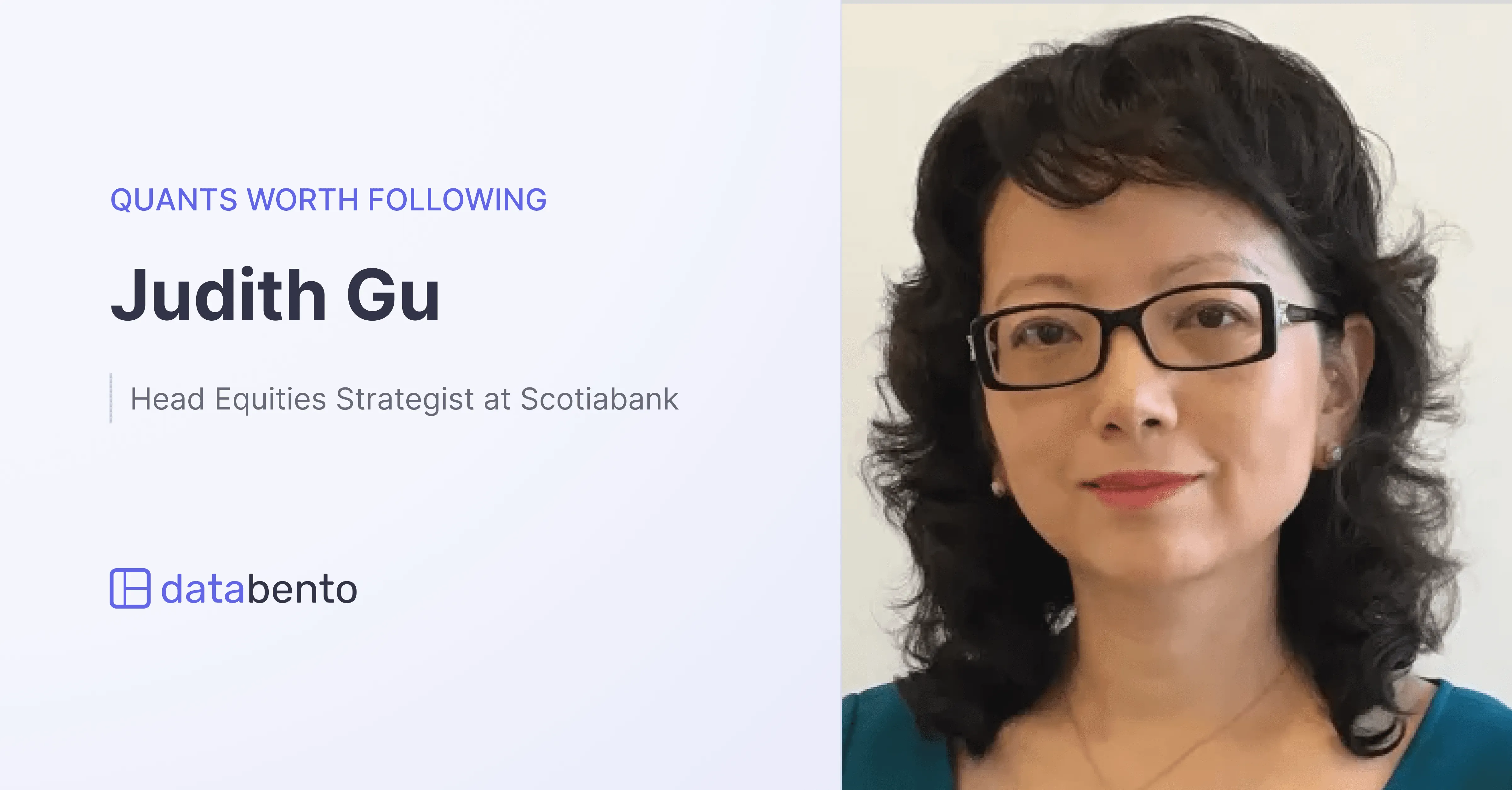
I actually did not go to a big name engineering school for college. I went to Russell Sage Women's College, a liberal arts college in a sleepy town called Troy, New York. And to me, the school was like my first family in this country.
"Quants worth following" is our latest interview series highlighting thought leaders in the quantitative trading industry who actively share their knowledge and resources with the community.
We had an insightful conversation with Judith Gu, Managing Director and Head of Quantitative Equities Strategists at Scotiabank. Judith oversees North American equities quant trading for both the US and Canada, specializing in data-driven, systematic real-time market-making, trading risk management, and alpha generation. Before joining Scotiabank, she was an equities strategist at Goldman Sachs, working in quantitative asset management and the equities market-making desk for 16 years, with a total of 25+ years in the quant trading industry.
Judith discusses how she entered the industry, sought-after job skills, finance podcasts to stay informed, and trends quants can expect from emerging technologies.
"I actually did not go to a big-name engineering school for college. I went to a liberal arts women's college in a very sleepy town called Troy, New York. The school I went to was Russell Sage Women's College. And to me, the school was like my first family in this country."
Judith shares her journey of moving to the US from Shanghai, China, to attend college for computer science and accounting. Despite not attending a well-known engineering school, her early career industry experience and continued education laid the foundation for her successful career.
"Post college, I started a technical job on Wall Street. Picking up from there, I eventually got a master's degree in finance and a master's degree in what we called Data Mining back then, but it was really modern-day data science.
That was twenty-plus years ago. And in statistics, I took off my quant career from there. So, I think the most surprising thing is that it wasn't a big engineering school background, but I ended up working a lot in the quant industry."
"My balance of day-to-day work is actually pretty well divided between research and collaborations with others, as well as looking at live cases of trading that are out of our expectations, like the outliers."
Judith illustrates her work routine as an effective feedback loop consisting of research, and internal and external collaboration to identify new research opportunities. This cycle enables her to keep innovating and adapting as the industry continues to evolve.
"There are days I can focus more time on research. So, that's probably more than about half of the week, which I consider pretty lucky to be able to focus on research and just go through the back task teams and think through the space. Other times, I spend on collaborating. So, collaborations with other researchers from other parts of the bank, not necessarily equities, but also fixed income and currency.
I also liaison with other outstanding data scientists from research firms and listening to new approaches, like, how do they solve a problem in a better way? And also looking at in our live learning systems, some of the outliers. How do we identify them and how do we actually catch them in the real-time space? It's a good feedback loop back into research."
When assembling a team, Judith prioritizes technical proficiencies and values collaborative personalities, highlighting the importance of a well-rounded skillset to succeed in quant trading.
"We definitely start with the hard skills in math and coding, these are the two major areas we focus on. The person has to be able to pick up good coding habits. It doesn't have to be fancy, but good coding habits, understanding a problem quickly, breaking it down to very scientific components, and responding in a reasonable way. Also, good mathematics and framework thinking. So, definitely these two hot skills. That's the first cut, for sure.
In addition to that, we look for collaborative personalities, openness, and a way of engaging and being passionate and curious about their work. We're looking for people who have an ownership mindset instead of 'I'm here to do a job; you tell me what to do.' Definitely, ownership so they can drive their project and drive the quality of their product."
"I'm going to borrow a line from Lisa Su, the CEO of AMD. She recently said, 'What skills are not going out of fashion? The skill always comes back to figuring out how to think on your own,' like how to think through a problem, how to be comfortable being alone and solving the problem on your own.
It may not be solely a mathematical problem; it may not just be storytelling. I think in the quant field, it's highly multidisciplinary. So, figure out your parameters, figure out the problem you're solving, and don't be afraid of making mistakes, but still solving it through. Don't be afraid of hitting barriers and not being able to solve it through in a day. Keep coming back and learning the skills that you need to learn and keep going. I think that will, hopefully, not go out of fashion with all the disruptive technologies we're having."
The biggest takeaway from Judith's advice is the adaptability and perseverance needed to prosper in the industry, along with the necessary critical thinking and problem-solving skills and the ability to find value in your mistakes.
"If I have to pick the top three, the first one is Flirting with Models with Corey Hofstein, the second one will be Excess Returns with Jack Forehand, and also Masters in Business with Barry Ritholtz.
These are the top three, but I occasionally listen to Lex Fridman. His podcast tends to be a bit long, but occasionally, I do listen to him. It's very enlightening. And I definitely start my day with Wall Street Breakfast."
Judith sheds light on the copious amount of content being produced in the industry on a daily basis, distinguishing between valuable information and noise to avoid. Reflecting on the past 10-15 years, she notes much of this content involves immense technological advancements.
"Machine learning is lots of good algos and faster processes, but still uses the basic techniques of optimization. But how do we actually position ourselves to keep learning through the disruptive technologies while also grounding us on basic fundamental skillsets and keep being solid on foundational skills? I think that's still the question for the next wave of quants."
She emphasizes the necessity of having a solid understanding of emerging technologies to effectively use them while ensuring that foundational skills are still at the center of decision-making.
"I think as quants, we'll be hearing a lot of other questions coming at us even faster and more frequently, such as why don't you use generative AI? Why don't you use deep learning? How do large language models help you solve your problems or select your features? Those questions are coming at a bigger speed and a large enormity of momentum. So, I think we just need to know what's actually going on internally with those technologies to be confident."
Check out the full interview on our YouTube here.