Quants worth following: Paul Aston
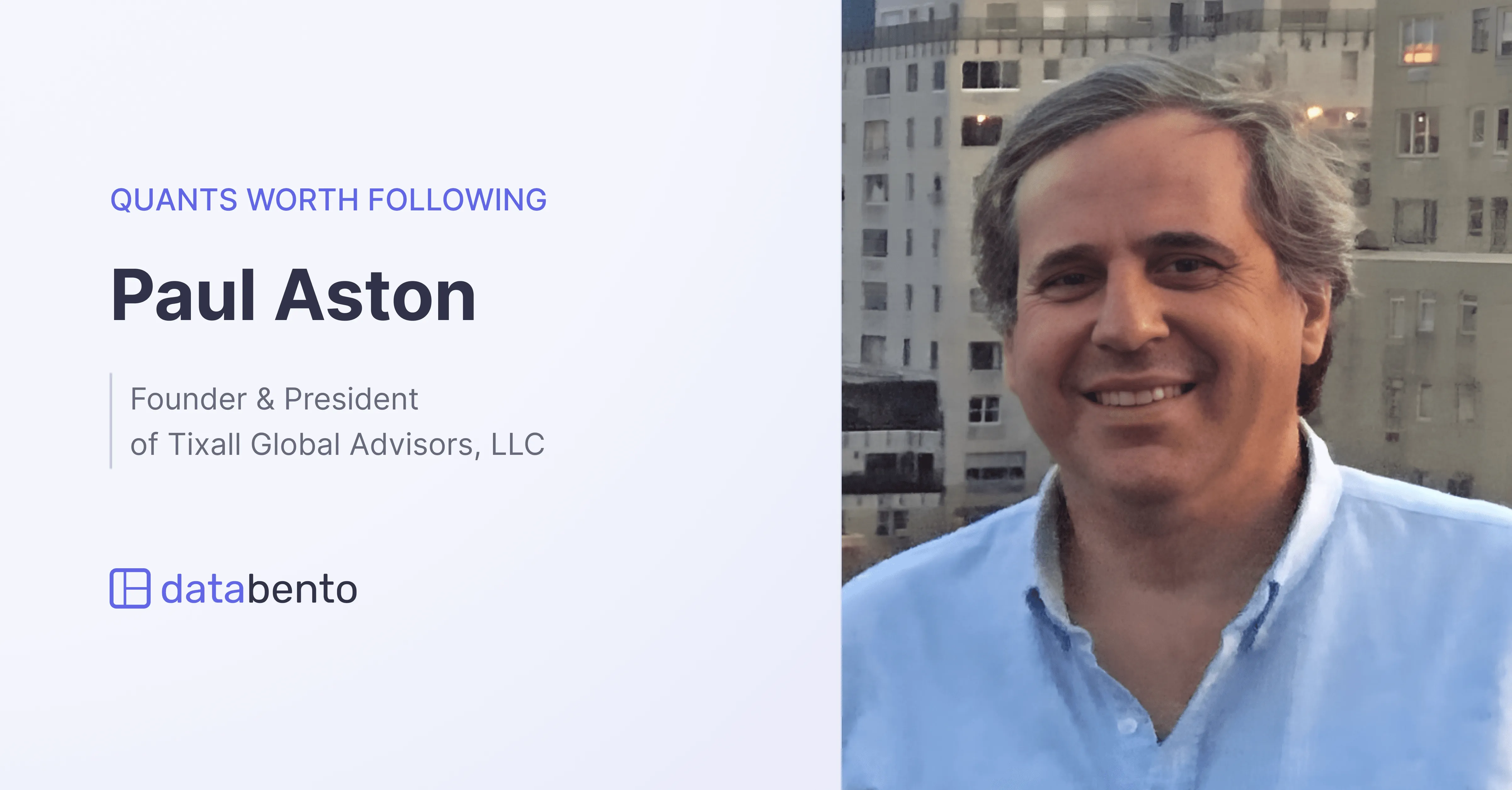
We weren't getting the expected alpha that our backtest showed. It just wasn't materializing. We didn't lose any money, but because that alpha was absent, it required us to reflect and ask why.
That spawned my deep dive into microstructure analysis, and it all came down to suboptimal implementation. Not having a deep understanding of liquidity flows, double auction markets, order books, and all of those phenomena can vastly affect the outcome of what is otherwise a medium-term or lower-frequency implemented model. That experience is a great example of how your career can evolve based on learning experiences and events along the way.
"Quants worth following" is our latest interview series highlighting thought leaders in the quantitative trading industry actively sharing their knowledge and resources with the community.
This week, we interviewed Paul Aston, the Founder and President of Tixall Global Advisors, LLC, an institutional portfolio management and quantitative advisory firm specializing in active alpha overlay, algorithmic trading, and statistical arbitrage strategies in global foreign exchange and other major asset classes.
Paul is also an industry leader with over three decades of experience on both the buy and sell sides. He has cultivated deep expertise as a quantitative strategist, portfolio manager, and trader across asset classes such as international equities, fixed income, foreign exchange, and derivatives.
Early in his career, he held positions at Baring Asset Management, Santander Global Advisors, Putnam Investments, Fleet/Columbia Management, and his equity statistical arbitrage fund, Prometheus Quantitative Strategies. He has also led the quantitative trading groups at major banks, including Bank of America, Morgan Stanley, and TD Securities.
In this interview, Paul shares his journey into quant trading, insights into the industry's evolution, his perspectives on AI, and the key lessons he's learned as both a quant and a business leader.
Paul discusses how his early studies in classical music composition and competitive sailing uniquely shaped his analytical approach, setting him on the path to quant trading.
"I didn't start as a quant. I started as a composer. In that art form, I was doing artistically relatively quantitative work, including a lot of combinatorial, information, and theoretic work.
I was also sailing competitively. I started trying to figure out how to gain an edge. In my spare time, I would start reading about the hydro aerodynamics of boats and things like that and start building those kinds of models. And then that started morphing into a more disciplined and analytic approach to doing things."
Eventually, after interning at Solomon Brothers during his first year of college, he earned a graduate degree in mathematical economics, marking his official entry into the markets with a focus on arbitrage.
Looking back on his career in the quant industry, Paul highlights the factors that have shaped modern trading into the data-driven field we see today.
"It's clear that our path was significantly influenced by the macro economy, crises, liquidity, and regulations. When I first entered the field, quants were primarily focused on derivatives pricing models, large-scale econometric models, and portfolio optimization. These strategies were more macro-scale, predicting larger investment opportunities.
As more crises started to pop up, risk and credit became bigger issues. Then, we entered this large-scale, long 20-year span of quantitative easing, where interest rates went to zero. That effectively meant simple carry yields went to zero, so there was no intrinsic yield left in markets. It became very speculative and volatility-driven, conducive to the rise of algorithmic and high-frequency trading. The only reliable way to predict alpha was through high-frequency liquidity flows, which never changed. They're always there, and they're always very self-similar.
That's where we saw quant trading take on a whole new realm and discipline. It evolved into the modern type of trading we see today, driven very much by data, systems, microstructure, and high-level data science-driven quant development skill sets. These skills existed before but were not in the same demand or form now."
Paul reflects on the surprising power of large language models (LLMs) like ChatGPT, drawing from his early experiences with the technology and observing how it has evolved.
"In the early 90s, when we were trying to read headlines, respond to headlines, and map that to liquidity and volatility spikes, we used tools to read streaming headlines and interpret what that might mean. But these language models have taken that to a far deeper degree. The technology and the ability to analyze and separate those features are much more robust now. So, deep learning, machine learning, and neural network technologies have evolved and become very powerful."
Despite these advancements, he expresses concerns about AI's application in trading:
"The big problem with AI right now is that it's tough to understand what and why it's doing is impressive. The outcomes and how all those connections are mapped to a solution are difficult to see inside that black box. The goal of trading, particularly in statistical arbitrage, is to eliminate uncertainty. AI still introduces considerable uncertainty into your equation, so its effectiveness has yet to be seen. However, it is a very powerful tool for filtering information and data that is otherwise difficult to come by.
Part of my ongoing research involves a lot of work with non-linear, non-stationary prediction. The big problem for AI is that I don't know if it does well in those types of environments. It can probably identify and handle turbulence, right? If you fed it wave tank information, you might be like, okay, what phenomena is happening here?
It could identify turbulent patterns and tell you something about them. However, predicting what types of things are happening in turbulence and noise would be very hard. Our markets are really dominated by noise. And where it's really strong, like in LLMs, language is very redundant."
Claude Shannon's book, The Mathematical Theory of Communication, also demonstrates how sentence and vocabulary structures can advance using information theory alone. "Many AI and LLMs exploit that structure in language, which is robust and redundant by its very nature. The big trick to applying it to our financial markets is to determine the syntax and redundancy in the financial markets that become predictable and exploitable."
As one of the leading quant traders in the field, Paul shares two key lessons he has learned throughout his career:
Break down your problem to understand what you’re trading, why you’re trading it, and what you hope to achieve.
"If I were to teach someone new to trading the core principles, the first thing I'd emphasize is understanding the definition of statistical arbitrage. There needs to be a favorable asymmetry between payoffs and odds. For example, if you’re betting on a coin flip with no predictability, a 50-50 chance, but you find that heads pays more than tails, you have a statistical arbitrage opportunity.
When approaching trades from this perspective, your focus as a quant isn't on predicting outcomes. Instead, it's about analyzing data to identify opportunities where you can achieve an asymmetric payoff. In other words, you seek situations where you are more likely to get paid for a particular outcome."
Success requires asset appreciation when long or depreciation when short. For statistical arbitrage to succeed, payoffs must be asymmetric and in our favor, needing a hit ratio above 50% with equal payoffs or correct directional payoffs exceeding wrong drawdowns.
"The fundamental models can be categorized as Boolean, binary, or negative binomial. These models focus on identifying a series of favorable outcomes and exploring them through permutations or combinatorial space. They serve as the basis for making predictions about your portfolio and positions.
As a quant, your task is to systematize this process by focusing on two essential aspects: understanding the payoff or cost associated with each event in your event space and determining the probability of that event or what information gives you an edge. You systematically explore these opportunities to identify favorable ones, which then become your bets.
However, it's important to realize that none of that is correct. You must ruthlessly manage risk and never overly bet on a weak hand. Adopt a disciplined approach, like playing poker, where survival depends on careful strategy rather than aggressive betting. Your focus should be on managing risk and making informed decisions rather than just chasing alpha. That's the only way you're going to survive the game."
"That happens all the time to a degree. Eventually, a good quant has to divorce themselves from the model they designed. In theory, all the systematic information should already be contained in your model and the process. That's what systematically happened. It should be very rules-based and rigorously applied.
When you step back from that, understanding how that works requires discretion. Discretion is where you have to use your spidey sense, macro sense, or risk sense for the intangible stuff that the human brain is good at understanding and the model is not. You have to be able to manage the noise."
There are also occasions where, despite favorable backtest results, strategies fail to perform in live markets. Paul describes a pivotal moment in his career when he decided to shut down his equity statistical arbitrage strategy at Prometheus Quantitative Strategies and return the funds to his investors.
"I wasn't getting the expected alpha that our backtest showed. It just wasn't materializing. We didn't lose any money, but because that alpha was absent, it required us to reflect and ask why.
That spawned my deep dive and expertise in microstructure analysis, and it all came down to suboptimal implementation. Not having a deep understanding of liquidity flows, double auction markets, order books, and all of those phenomena can vastly affect the outcome of what is otherwise a medium-term or lower-frequency implemented model. That experience is a great example of how your career can evolve based on learning experiences and events."
Throughout his career, Paul has led both Tixall Global Advisors and Prometheus Quantitative Strategies. Here are two key lessons he's learned along the way:
"Every company has customers or clients. Even if you're trading your own capital, you must understand the industry's pain points and needs. If you don't know that, you're in trouble because nothing you'll do will solve it. So, you need to understand the customer's pain point and deliver succinctly and uniquely with an advantage.
And then there's the chicken and egg problem in starting a business. Is my customer's pain points something I'm already really good at? Or is that something I need to become good at or evolve? Something that often happens is that quants or even eager entrepreneurs dive into something they are passionate about. They might have a genuine interest in this and might not be good at it, but they dive in. Or they might be good at it as well, but they don't know the customer base yet, and all they end up doing is spinning their wheels because the revenue model, the argument, or the application is missing.
Going back to that fundamental principle of statistical arbitrage. What's the payoff? They could have a great product, and everything comes out nicely, but you don't have a business if the payoff is not associated with it. There's no income, revenue, earnings, or gain in the system."
"Be ruthless in having contracts and communicating things up front. If anybody balks at communication, clarity, and putting things in writing, then you absolutely cannot engage in those types of relationships. There will always be a point of contention, and that will become, at best, distracting and, at worst, force you out of business. So it's important to ensure everything is highly systematic and contractual, and then you must ruthlessly manage a budget.
You cannot waste time and money on lawyers, vendors, accountants, and systems. You know, you can't spend too much. You should spend your time trying to do as much of that yourself as possible, even though that's not the most efficient way. But you have to spend time trying to do it yourself because until you've done it yourself, you don't know what's involved. You don't see the value of what somebody else can deliver who's better at it than you are. And then you also don't know the appropriate price tag for that stuff."
"As we continue to develop our business, perfecting our active alpha overlay is a major focus. We hedge foreign exchange for institutional investors, which is costly and not always straightforward. Our alpha strategies substantially lower the costs of hedge implementation, collateralization, and putting on and rolling transactions.
We combine this with active alpha, alpha-generating pure hedge fund strategies to ensure those costs come down. You could even generate diversification and an independent form of returns for the program. So we're building that out, perfecting the systems behind it, with live capital and a live client in size.
We aim to grow those assets and that responsibility to a respectable size of a billion plus. That's the game plan over the next couple of years. Then, from there, there are all kinds of things that spill off of there that we could take advantage of.
If you have that competency, you can move into offering liquidity and doing things like that as a separate business to your customers. In the market in general, there are specialized analytics and systems that are nonproprietary and part of our overall process that could be spun off."
Check out the full interview on our YouTube here.