Quants worth following: Vinesh Jha
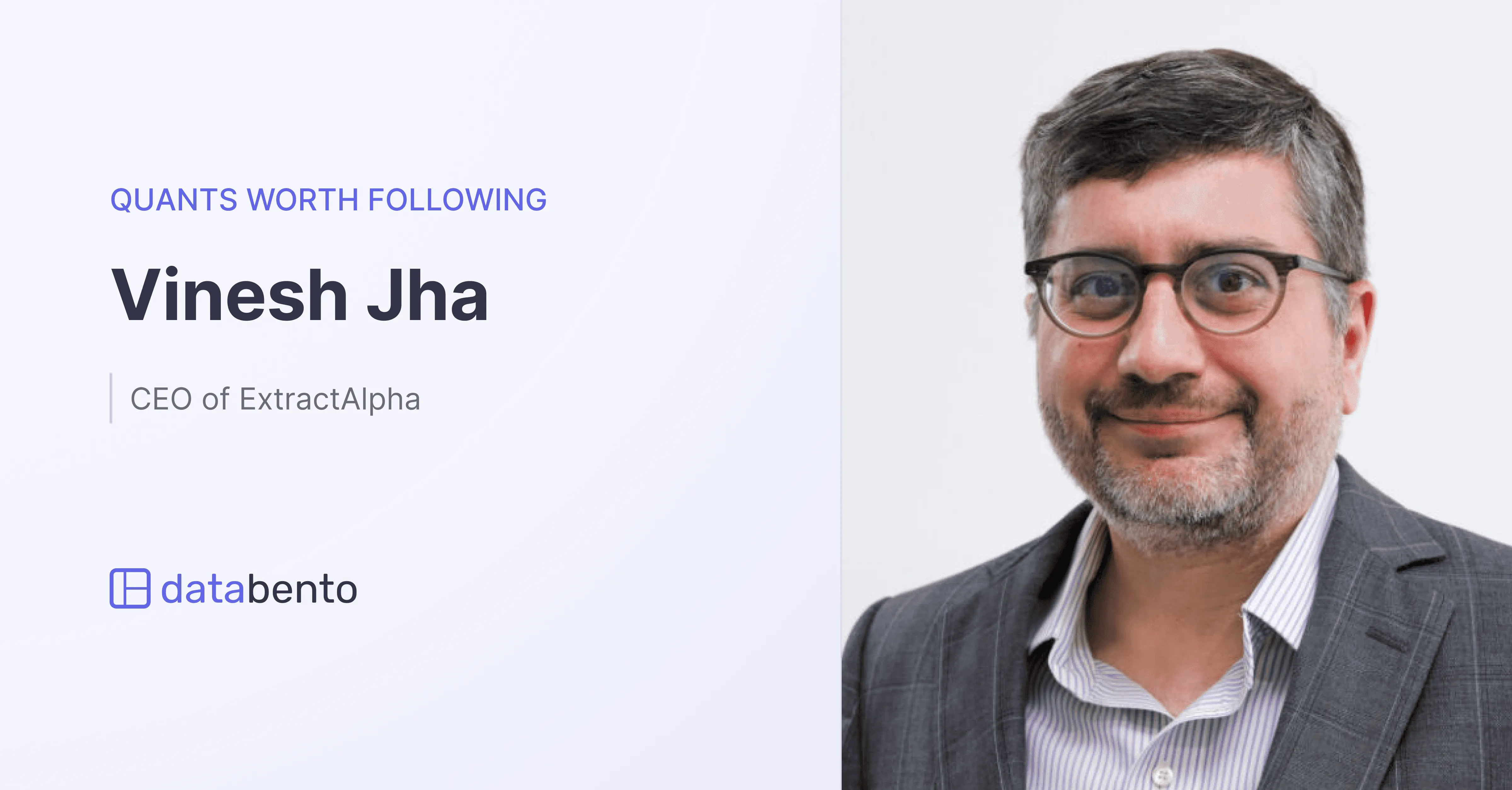
"Quants worth following" is our latest interview series highlighting thought leaders in the quantitative trading industry actively sharing their knowledge and resources with the community.
In this edition, we sat down with Vinesh Jha, the founder and CEO of ExtractAlpha. Vinesh launched the firm in 2013 with the mission of bringing analytical rigor to the development of alternative datasets and signals for the capital markets. ExtractAlpha also owns and operates Estimize, a crowdsourcing platform that predicts earnings for equities.
Earlier in Vinesh's career, he was the Director of Quantitative Research at StarMine, and subsequently, he developed systematic trading strategies for proprietary trading desks at Merrill Lynch and Morgan Stanley. He was Executive Director at PDT Partners before starting ExtractAlpha. His team now stretches across the US, Europe and Asia, and they offer ~16 different datasets to quant trading teams at financial institutions and hedge funds worldwide.
Vinesh delves into the origins of ExtractAlpha and the biggest lessons he's learned from it. He discusses the distinctions between working in quant trading and the data world, dealing with trends in the industry and his own predictions, and the importance of making mistakes in your career.
Vinesh describes his unexpected transition into the data industry brought upon by a non-compete and the desire to build his own company.
"Early in my career, I was on the data side; StarMine was a data company. So, I've seen both sides of the industry, and knew what I was getting into when I went into the data world. There are pros and cons to each of these worlds, but what made me get into it was nothing more than a non-compete.
Essentially, I had left PDT, which was a spin-out of Morgan Stanley. Both when I left StarMine and PDT, there was something about this sort of Dunbar's number concept of these groups that reached 100-150 people. I kind of didn't know everybody. It didn't feel like a startup anymore, and it didn't feel as entrepreneurial. So I wanted to do something more on my own, and I fell into the data world again."
Vinesh didn't initially set out to start a data company. ExtractAlpha came about during his time as a consultant.
"We didn't really have a plan; the company sort of sprung up out of nowhere. I was doing some consulting with data companies who needed help figuring out how to monetize their data, and it sort of morphed from there into a data company."
Those in the market data sector and the quant trading industry work toward different goals. Vinesh speaks to the distinctions between the two, and more specifically, in his case, the differences that come with starting a data company.
"They're very different day-to-day, as you can imagine, I'm not worrying about a PnL all the time. I would say there are highs and lows for both, but they're more attenuated in the data world. We’ve built a data-as-a-service business – something offering enterprise value and something in the long term that pays off, whether it's dividends or it gets acquired or whatever it is. It's more than the day-to-day worrying about PnL."
ExtractAlpha has been around for over a decade, outlasting other data companies trying to make it. Here are two lessons Vinesh has learned running ExtractAlpha:
"If you can maintain control of your company and not take too much in outside investments, it's great to do it that way if you have the stomach for it. I don't always have the stomach for it, but I generally think it's a good way to go if you can. The downside to that is everything you want to do takes 10x as long, it's 10x harder, and you have to do it yourself. You have to do all the things you're not comfortable doing."
"It takes a long time to get the right pieces in place, and I think we're there now. We have a great research team, sales and marketing team, and operations team. Everything's kind of there and humming, but that was not the case for a long time. You've got to do absolutely everything yourself in the early days. Everything from going down to the bank, opening the bank account, and doing stuff you never think about. You have to make sure that everything happens. So I think you have to be ready for that if you're going to start something like this, especially for the long haul."
At ExtractAlpha, there are three main themes of datasets: earnings-related, natural language processing, and Asia-specific datasets. Vinesh explains why they decided to pursue each of these areas.
"There are a few different themes that we've supported. We're developing datasets that we think are going to be valuable and additive to an active quant process. We have certain strengths we've developed over the years – they are themes around earnings-related data, natural language processing (NLP), and Asia-specific datasets. Not everything we do falls into these buckets; some of them are in-between buckets, but those are generally the themes. Part of that is, I have a lot of background personally in earnings-related data. We have a dataset called TrueBeats with predictions of earnings surprise, and that's related to the work I used to do back at StarMine."
"Our research team has focused a lot on NLP in the last couple of years, and not surprisingly, a lot of people are doing that. We have a very popular dataset that looks at extracting value from earnings call transcripts, for example. And we're starting to extend that to news data. We have NLP products not just for English but for Japanese and Chinese, so for multiple languages based on different corpora."
"We have a couple of datasets that focus on Asia. We're headquartered in Hong Kong, and we have native Chinese speakers on our staff. So we've developed a bunch of expertise in local datasets. Some of these are based on language, but some of these are not. We're looking at point-of-sale data for Japan; we're looking at earnings call transcripts for China. We're looking at really unique datasets that we think people will be interested in when they're looking for something to build out their Asia exposure and diversify their alpha streams."
Vinesh explains that as researchers, his team is naturally skeptical and approaches everything cautiously. After looking at dozens of datasets yearly, he often finds little value in most of them. However, when he does, it comes from odd areas.
He shares his experience with finding predictive value in ESG data. "I can talk about ESG specifically because it's a very fragmented space in a lot of senses. There's no sort of core ground truth really, but we actually have found some value in it." He demonstrates how to find the sentiment in news data related to ESG: "Part of that is taking news data, trying to find ESG-related topics in it, tagging it to both companies and topics, and then finding the sentiment in that news. If you're doing it that way, then you really have an outside-in view of a company's ESG exposures that are not generated from the company's own reports. Then, it's sort of a variant on news sentiment, which is something that's well-known. It's not surprising that we found value in news sentiment, but it's news sentiment with an ESG twist."
Despite the excitement of the latest trends and advancements, sometimes simplicity is more effective. Vinesh noted, "We'll look at things that are topical. We've looked at large language models and so on, and try to apply them and sometimes simpler is better. Sometimes the latest thing, I don't want to call it a fad, but something simpler can fit the bill and can accomplish what you need in terms of predicting returns as opposed to the latest and greatest."
Vinesh explores three niche areas that ExtractAlpha covers: Chinese equities, sentiment signals from independent researchers, and clickstream data from financial websites.
"We do have quite a few datasets that cover Chinese equities. I think the long view here is that China is sort of a deep and liquid market, and it's going through growing pains, right? And this is going through growing pains both from the perspective of the quants, who have been bold enough to enter that market, and they're mostly domestic funds. But also the regulators are going through growing pains and trying to figure out how to regulate this new thing which is quant in China. So we have a bunch of datasets that cover China from various aspects."
"We've got a really interesting dataset where we've collaborated with a company in Singapore called Smartkarma. Smartkarma is essentially an independent research provider network. It's a place where you can, if you're a fundamental investor, go and read research reports from non sell-side researchers in Asian companies, which is a really great resource. But in addition to that, these research reports are data. So we've taken those and turned them into the IRP Sentiment Signal, a signal based on reports from independent researchers."
"We have access to a dataset with clickstream data from financial websites, and created a Digital Revenue Signal. If you want to know who's looking at the Apple page, the NVIDIA page, on the financial sites for retail investors, we track that through the ad sales and cookie tracking. So it's an interesting way to get a view of who's looking at what. And what that really teaches you, in addition to just knowing what's really popular for retail investors, is which stocks are inefficiently priced. It's a really interesting way of layering on a view of which segments of the equity market might be better fishing grounds for quant strategies."
Vinesh suggests not only having a solid understanding of the industry's ecosystem, but going beyond the academic courses and literature to gain hands-on experience with personal projects.
"Have a good grounding on corporate earnings and how they work; on how the ecosystem works between the sell-side and the buy-side. And corporates on the traditional behavioral finance research literature, as well. Understanding how all those things fit together."
Perhaps more important than reading about industry knowledge is gaining experiential knowledge. Vinesh states, "If you're starting out, you have to get your hands dirty with a project more than anything else. This may be obvious, but going beyond the courses. You have to pick something out that you're passionate about and try it. You're going to screw it up, and you'd rather screw it up on your project than on someone else's project."
He advises exposing yourself to making mistakes and the benefits that come with it, especially earlier in your career. "Typically it's something like lookahead bias or something like that. You didn't lag the data correctly. Something simple, but those are going to be important lessons. And if you get those lessons early, it's going to help you. It'll become second nature when you're doing research later on."
"We've seen this move towards things getting more quantitative, systematic, with data really driving things forward. I think that will continue. We've seen that it's reached a point of maturity in something like US equities or macro. That's how a lot of investors behave now, and a lot of data scientists and quants in the world are pursuing those things in those markets."
A few trends Vinesh predicts will continue to surface are emerging markets and asset classes. "I mentioned China, that's a good example. Other emerging markets like India I think will be similar. Also other asset classes. I think with something like crypto, people very quickly approach it with a quantitative view, but there's less fundamental data there."
Throughout his career, Vinesh has seen a pattern of people believing that fundamentals didn't matter. When he was first starting out, people talked about the insignificance of earnings and to value a company on eyeballs. He mentions:
"Those dislocations maybe feel like they're happening more often, but they've always happened… Since they're dislocations, there will be a reversal back to normalcy at some point. So there's always going to be value in predicting something really fundamental. In the case of stocks, that's why we put so much emphasis on forecastings, earnings, revenues, company fundamentals, and cash flows."
Watch the full interview on our YouTube here.